Does your organization struggle to provide a return on investments in digital analytics?
Many organizations collect and report digital analytics data from their websites, but unfortunately, they often fail to derive insights and take action based on those insights.
This results in missed opportunities and a lack of business impact.
It’s time to transition from the outdated CRAP strategy to the more effective CARE framework and, indeed, level up your digital analytics game.
Let me start with an explanation of CRAP and CARE strategies.
Understanding the CRAP Process
Most companies worldwide follow the traditional CRAP strategy. It includes four stages:
- C – Collect Data
- R – Report Data
- A – Avoid Analysis
- P – Postpone Action
Collect Data
Data collection (C) is at the core of the CRAP strategy. Google Analytics (or a similar tool) is installed on the site and appears to collect data more or less reliably.
However, the setup is rarely planned or documented, leading to varying data quality. The reliability of the data is not ensured through audits, nor is it monitored regularly.
Because of this, the stakeholders don’t trust the data. For a good reason.
Report Data
Data is primarily used for reporting (R).
Basic analytics metrics are reported weekly, month after month, answering questions like “How many?”.
Fortunately, manually compiled reports have been replaced by automatically updating dashboards, saving work and reducing copying errors.
Yet, these reports often rely on incomplete or incorrect data, which can lead to new problems if and when the data is used for decision-making.
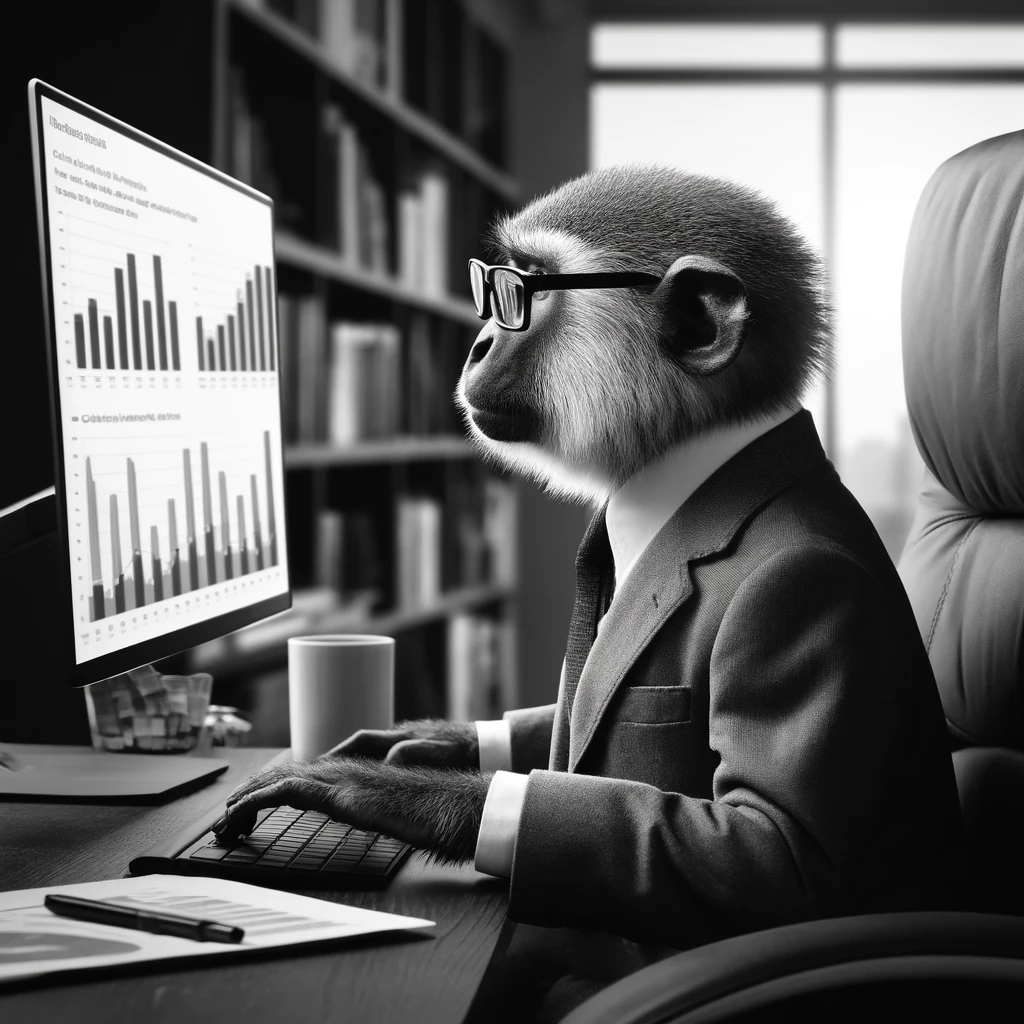
Avoid Analysis
The third stage brings some relief. Avoiding analysis (A) allows marketers to breathe a sigh of relief.
The report has arrived in the email inbox, so there is no need to ponder the numbers anymore.
PDF files gradually fill the inbox, from which they are eventually cleaned out to free up space.
Occasionally, numbers are discussed in board meetings but quickly dismissed as irrelevant or untrustworthy.
Postpone Action
The endpoint (P) is simple: avoid changing the current state based on data-driven conclusions.
By not proposing significant changes, one does not take major risks. Marketers are, after all, risk-averse like most other people.
If changes are needed, they are based on intuition rather than analytics data, which is often not trusted.
In the worst cases, decisions are made based on the highest-paid person’s opinion.
Introducing the CARE Framework
There is an alternative to CRAP analytics: the CARE framework.
Unfortunately, few companies use this framework, even if some more famous analytics professionals have mentioned it.
The CARE framework consists of four stages:
- C – Collect Data
- A – Analyse Data
- R – Recommend Actions
- E – Experiment and Execute
Collect Data
Collecting data is an essential step in digital analytics. This involves setting up tracking and reporting mechanisms.
Ensuring that your data is accurate, relevant, and compliant during this phase is crucial.
- Accuracy: Data should be as precise as possible. Automating quality assurance for tracking data is recommended. Automated tests can help identify issues related to consent management.
- Relevance: Data collection should align with business goals. For instance, if your goal is to increase online sales, relevant metrics might include conversion rate, average order value, and revenue per session.
- Compliance: Compliance with privacy legislation is critical when we CARE. You should not collect data you don’t need; you should follow the principle of data minimisation, store it only as long as required, and have a legal basis for using data. In short, don’t be a jerk.
Accurate and relevant data helps identify opportunities to optimize your website and marketing campaigns. Compliance minimizes your legal and brand risks.
Analyse Data
Collecting data is only the beginning; you need to analyze it to derive insights. The word analysis comes from the Ancient Greek ἀνάλυσις (analysis, “a breaking-up”). So, we need to break up the metrics into smaller parts to understand them better.
- Investigate Changes: If your conversion rate decreases, find potential causes. If the average order value rises, identify the drivers behind this increase.
- Identify Patterns: For example, look for products with better or lower-than-average add-to-cart ratios. Identify differences between traffic sources and customer groups.
- Understanding Biases: Recognize biases caused by users’ lack of consent to tracking. Don’t simply assume that consenting users are a representative sample of all users. Additionally, understand the differences between your data sources and their idiosyncrasies.
Segmenting the data by different dimensions, such as traffic source and device type, allows you to find interesting patterns. The analysis phase involves using various tools and approaches:
- Data Visualization: Creating dashboards in tools like Looker Studio or Power BI helps track KPIs and identify patterns.
- Statistical Analysis: Tools like R or Python can help you dig deeper into the data.
- Predictive Analytics: Predictive analytics with machine learning algorithms can also help to predict future marketing outcomes.
Machine learning SaaS tools might replace some statistical programming tasks in the future. Currently, analysts need to analyze the data manually and provide actionable insights.
On the other hand, for most companies, simple data visualisation methods offer enough low-hanging fruits before investing in more advanced methods.
Recommend Actions
Once you’ve derived insights from your data, it’s time to recommend actions. This is critical for your analytics process.
- Website and Marketing Changes: Suggest optimizations for your website and marketing campaigns.
- Adjust Targeting and Messaging: Recommend adjustments based on data insights.
- Explore New Opportunities: For example, if many users drop off at a certain point in the checkout process, recommend optimizing that step.
As an analyst, you need to help make decisions. You won’t be the only decision-maker. In most cases, you are not a decision-maker, just a source of data and analyses.
so it’s essential to effectively communicate your ideas to other stakeholders.
Experiment and Execute
The final step in the CARE framework is to experiment and execute the recommendations.
- Testing Methods: A/B testing and sequential testing to validate your recommendations is the final moment of truth. And, of course, to test the highest-paid person’s ideas, too.
- Collaboration: Analysts should work with other teams, such as growth, PPC, and front-end teams, to implement experiments. It is rarely a good idea to do everything by yourself.
For instance, in e-commerce you could experiment with
- the checkout process to improve conversion rates
- the product pages to enhance add-to-cart rates
- the messaging to improve conversion rates.
Analysts should also assess the experiment’s results to determine whether their recommendations were effective and to improve the CARE process.
How to CARE more?
The CARE framework offers a structured, strategic approach to digital analytics. It ensures:
- Accurate data collection
- Creation of meaningful insights
- Provision of actionable recommendations
- Execution and assessment of experiments
You can improve customer experience, marketing, and website performance with CARE. It also helps enhance internal processes and demonstrate the value of analytics to key stakeholders.
Using CARE is an ongoing process, not a one-time implementation or change project.
Therefore, organizations need a dedicated analyst (either internal or external) to support decision-making. It’s also essential to involve analysts with communications, advertising, product development, and UX projects.
This sounds like a lot of work, and it is.
But it will make analysts’ work much more enjoyable and exciting than it ever was.